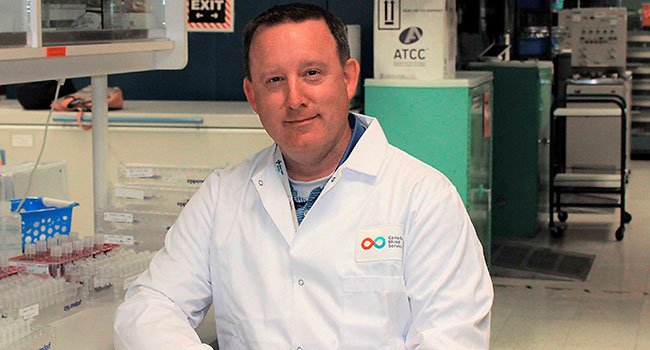
Jason Acker was a lead author of a new international study showing that machine learning can be used to analyze samples of red blood cells faster and more precisely than human experts – a finding that could change how donated blood is assessed, stored and selected for transfusion into patients. (Photo: Faculty of Medicine & Dentistry, August 2020)
Machine learning could change the way donated blood is evaluated for quality and selected for transfusion to patients, thanks to an international study that analyzed changes in the shape of red blood cells from stored samples.
The study, published in the journal Proceedings of the National Academy of Sciences, was a collaboration of experts in five countries and 12 academic institutions, including the University of Alberta.
“This project is an excellent example of how we are using our world-class expertise in precision health to contribute to the interdisciplinary work required to make fundamental changes in blood diagnostics,” said Jason Acker, professor in the Department of Laboratory Medicine & Pathology and one of the lead authors of the study.
Acker, also a senior scientist at Canadian Blood Services’ Centre for Innovation and a member of the Women and Children’s Health Research Institute, has decades of experience in the assessment of donated blood products.
“Canadian Blood Services is responsible for managing the blood supply in all provinces and territories except Quebec, and we’re chiefly concerned about the quality of the products. One of the things we routinely evaluate is the form of the cells, which we’ve been monitoring for 20 years using very traditional methods,” he explained.
The shape of things to come
When blood is taken outside the body and separated to be stored, red blood cells start changing their shape as they age, which eventually affects their ability to function and carry oxygen through the body’s tissues when they’re transfused. The red cell products can only be stored for 42 days, so they need to be monitored closely.
Currently, donated red blood cells are evaluated by placing a drop of blood on a glass slide and looking at the cells, classifying them based on their shape from a sample of 100 cells. A morphology index is calculated, which is a score of the average shape of the sample cells.
“It’s very time-consuming. We’re only looking at 100 cells, and it’s very subjective. One technician may give a score of 70, while another may give a score of 80,” said Acker. “It’s really important that we get it right, because we’re trying to monitor the progress of a product that will actually be transfused into Canadians.”
Looking to develop a faster and more accurate procedure to monitor the donated blood, Acker and his colleagues tapped into the potential of artificial intelligence. They used imaging flow cytometry – technology available at a few of the academic institutions involved, including the U of A – to capture images of tens of thousands of cells from a droplet of blood and create a large database for analysis.
With these images, the team was able to automate the traditional expert assessment by training a computer with example images of healthy and unhealthy red blood cell shapes. The automated process analyzed more than 100 blood samples – which usually takes months for a team of technicians – in just one day.
In addition to successfully replicating the traditional process, the researchers wanted to address the discrepancies between experts’ evaluations of the shapes of the cells.
“We said, ‘What happens if we don’t tell the computer what a sphere is?’ And we basically let it look at a variety of different parameters. That’s the nice thing about machine learning – it looks at things that we as humans would not even think about, and it generates data on that,” said Acker.
“The computer actually did a better job than we could, and it was able to pick up subtle differences in a way that we can’t as humans. It’s not surprising that the red cells don’t just go from one shape to another. This computer showed that there’s actually a gradual progression of shape in samples from blood products, and it’s able to better classify these changes. It radically changes the speed at which we can make these assessments of blood product quality.”
The successful outcome of the experiment provided the research team with algorithms that help show how machine learning can be used to classify the quality of red cells faster and more precisely. These algorithms are now used to study other factors that can affect the quality of donated blood products.
“This will help us identify donor factors such as age and gender, blood product manufacturing processes and storage conditions that we can focus on to make sure that we’re getting safe products to patients,” said Acker.
Opening doors to personalized transfusions
Among the other factors being identified, the technology was able to distinguish subpopulations of cells within the same blood product, which could help health professionals spot potential health issues faster and discover risk factors of certain products going to a specific patient.
Acker’s research is focused on learning whether there are potential risks of a patient receiving blood from a donor of the opposite sex, one example that would help categorize products and apply transfusions beyond the traditional classifications.
“We already match for different blood groups. We also select specific blood products for treatment of babies or for other patient groups,” explained Acker. “But what this research is leading us to is the fact that we have the ability to be much more precise in how we match blood donors and recipients based on specific characteristics of blood cells. Through this study we have developed machine learning tools that are going to help inform how this change in clinical practice evolves.”
| By Laura Vega
The views, opinions and positions expressed by columnists and contributors are the author’s alone. They do not inherently or expressly reflect the views, opinions and/or positions of our publication.